模板匹配
介绍
所谓模板匹配就是找到原图像上与模板图像相似的部分。
模板在原图像上从原点开始滑动,每一步都计算差别程度,计算方法有 6 种,参见 TemplateMatchModes_OpenCV docs:
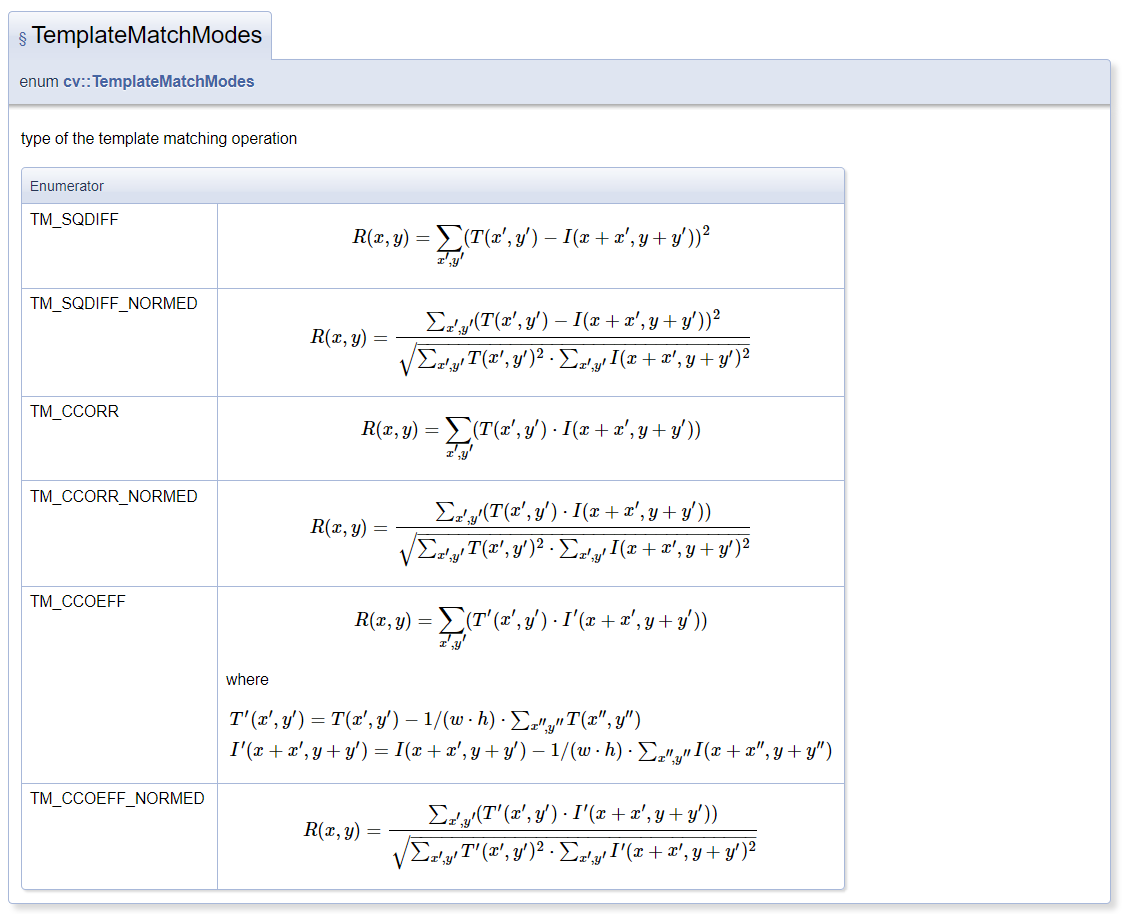
然后将每次计算的结果放入一个矩阵里,作为结果输出。
假如原图形是 AxB 大小,而模板是 axb 大小,那么容易知道输出结果的矩阵是 (A-a+1)x(B-b+1) 大小。
对这个输出矩阵使用 minMaxLoc() 函数,就可以得到矩阵中最大值、最小值的位置,也就对应于原图像的位置,就很容易知道是哪里匹配度最高了,就可以圈出来 ROI 了。
例
img = cv2.imread('media/lena.jpg', 0)
template = cv2.imread('media/face.jpg', 0)
h, w = template.shape
methods = ['cv2.TM_CCOEFF', 'cv2.TM_CCOEFF_NORMED', 'cv2.TM_CCORR',
'cv2.TM_CCORR_NORMED', 'cv2.TM_SQDIFF', 'cv2.TM_SQDIFF_NORMED']
for meth in methods:
img2 = img.copy()
method = eval(meth)
res = cv2.matchTemplate(img, template, method)
min_val, max_val, min_loc, max_loc = cv2.minMaxLoc(res)
if method in [cv2.TM_SQDIFF, cv2.TM_SQDIFF_NORMED]:
top_left = min_loc
else:
top_left = max_loc
bottom_right = (top_left[0] + w, top_left[1] + h)
cv2.rectangle(img2, top_left, bottom_right, 255, 2)
plt.subplot(121), plt.imshow(res, cmap='gray')
plt.xticks([]), plt.yticks([])
plt.subplot(122), plt.imshow(img2, cmap='gray')
plt.xticks([]), plt.yticks([])
plt.suptitle(meth)
plt.show()
模板:
匹配结果:
左边图中最亮或者最暗的地方就对应着最佳匹配区域。
匹配多个对象

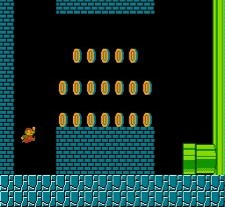
img_rgb = cv2.imread('media/mario.jpg')
img_gray = cv2.cvtColor(img_rgb, cv2.COLOR_BGR2GRAY)
template = cv2.imread('media/mario_coin.jpg', 0)
h, w = template.shape
res = cv2.matchTemplate(img_gray, template, cv2.TM_CCOEFF_NORMED)
threshold = 0.8 # 取匹配程度大于%80的坐标
loc = np.where(res >= threshold)
for pt in zip(*loc[::-1]): # *号表示可选参数
bottom_right = (pt[0] + w, pt[1] + h)
cv2.rectangle(img_rgb, pt, bottom_right, (0, 0, 255), 2)
cv2.imshow('res', img_rgb)
cv2.waitKey(0)
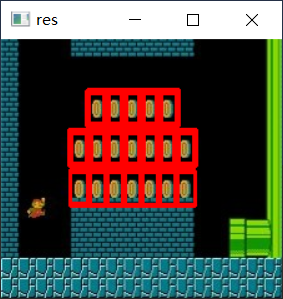
直方图
基本使用
直方图是一种统计用的图,对于图像,就是用于像素点的统计。
直方图中,横坐标是 0~255,纵坐标是个数。
cv2.calcHist(images, channels, mask, histSize, ranges)
images
:通常为灰度图,或者对图像 BGR 中的某一个通道进行统计。要用中括号[]
括上,如[img]
channels
:如果入图像是灰度图,该值就是[0]
;如果是彩色图像,该值可以是[0]
、[1]
或[2]
,分别对应 B、G、Rmask
:图像的掩模。如果统计整幅图像的直方图就设为None
histSize
:BIN(直方图中的每个长方体)的数目。也要用中括号ranges
:像素值范围,常为[0, 256]
img = cv2.imread('media/yuki.jpg', 0) # 0表示灰度图
hist = cv2.calcHist([img], [0], None, [256], [0, 256])
plt.hist(img.ravel(), 256)
plt.show()
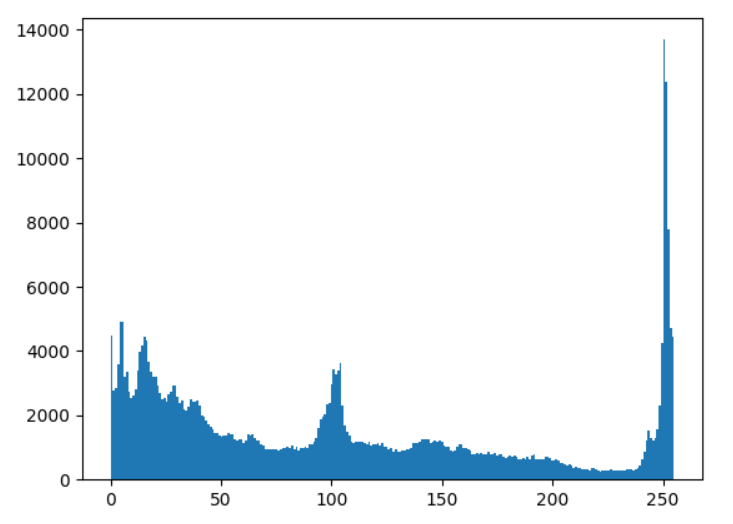
img = cv2.imread('media/yuki.jpg')
color = ('b', 'g', 'r')
for i, col in enumerate(color):
histr = cv2.calcHist([img], [i], None, [256], [0, 256])
plt.plot(histr, color=col)
plt.xlim([0, 256])
plt.show()
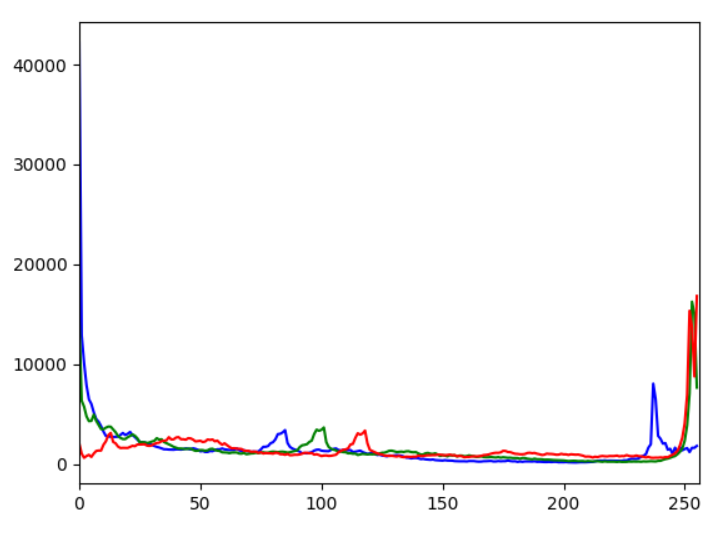
mask 的使用
img = cv2.imread('media/sky.jpg', 0)
mask = np.zeros(img.shape[:2], np.uint8)
mask[50:500, 300:600] = 255
masked_img = cv2.bitwise_and(img, img, mask=mask) # 与操作
hist_mask = cv2.calcHist([img], [0], mask, [256], [0, 256])
plt.subplot(221), plt.imshow(img, 'gray')
plt.subplot(222), plt.imshow(mask, 'gray')
plt.subplot(223), plt.imshow(masked_img, 'gray')
plt.subplot(224), plt.plot(hist_mask)
plt.xlim([0, 256])
plt.show()
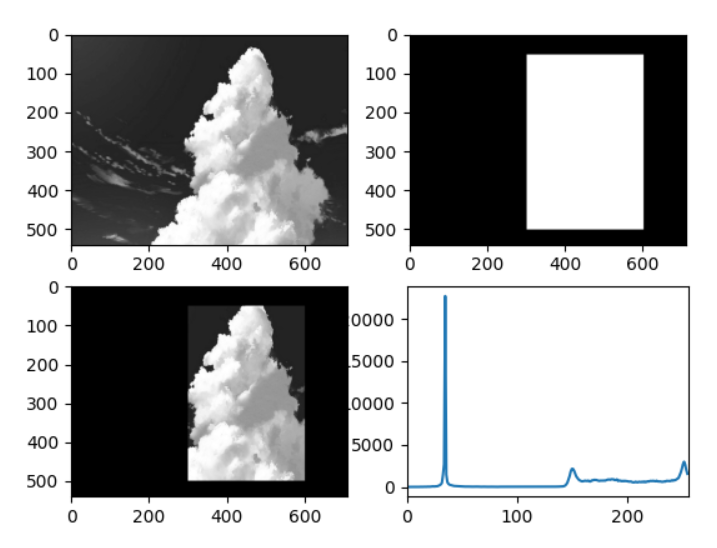
均衡化
一些图片的直方图分布可能是很不均匀的,也就是很 “瘦高”,直方图均衡化就是让直方图变得均匀一些,也就是 “矮胖” 一些。
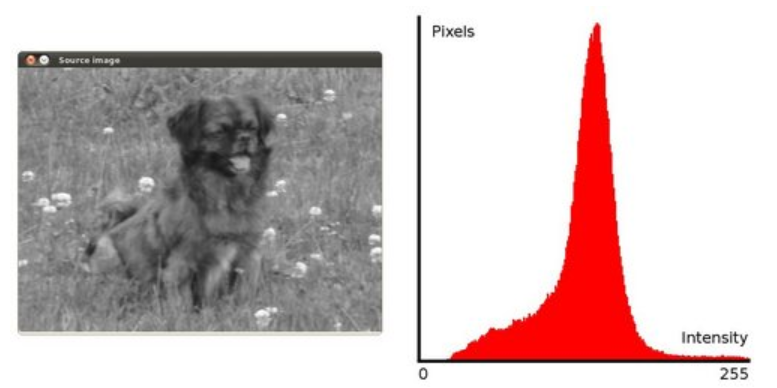
均衡方法示意:
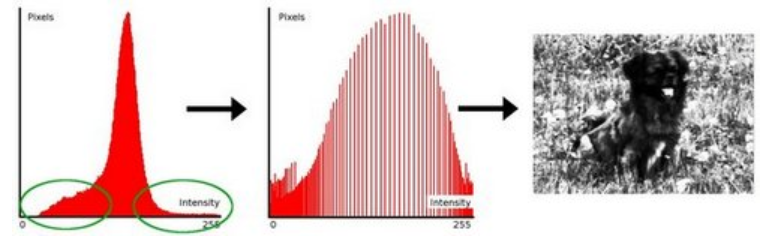
例:
img = cv2.imread('media/clahe.jpg', 0)
plt.hist(img.ravel(), 256)
plt.suptitle("origin")
plt.show()
equ = cv2.equalizeHist(img)
plt.hist(equ.ravel(), 256)
plt.suptitle("equ")
plt.show()
res = np.hstack((img, equ))
cv_show('origin, equ', res)
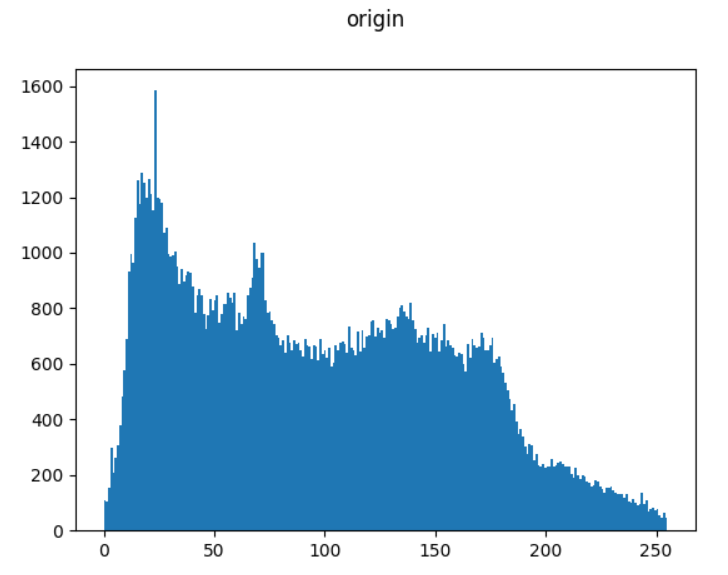
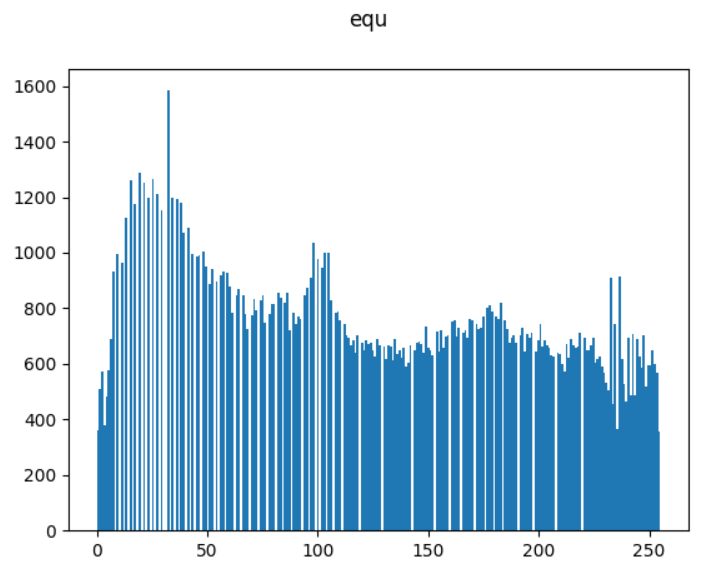
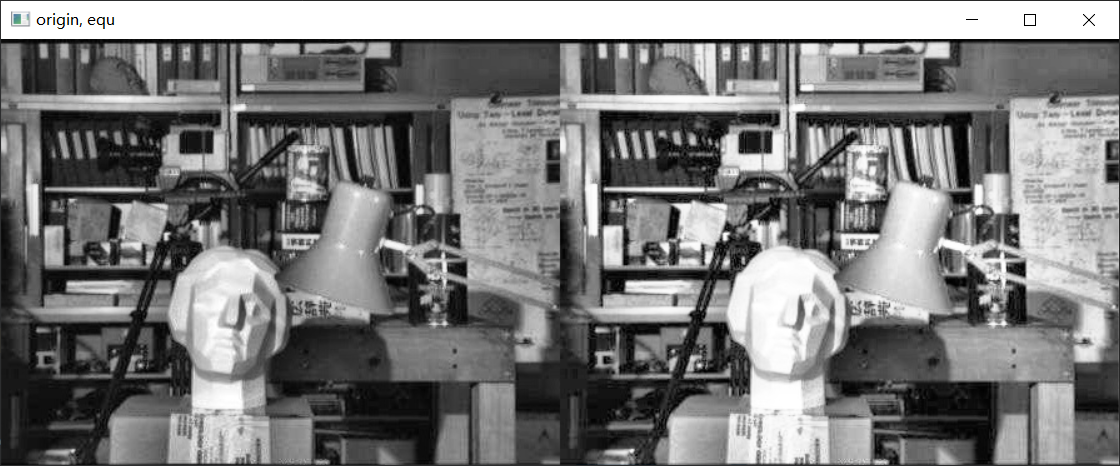
自适应均衡化
相比于上述的 cv2.equalizeHist
均衡化方法,自适应均衡化方法 cv2.createCLAHE
更适合于改进图像的局部对比度以及获得更多的图像细节。
img = cv2.imread('media/clahe.jpg', 0)
equ = cv2.equalizeHist(img)
clahe = cv2.createCLAHE(clipLimit=2.0, tileGridSize=(8, 8))
res_clahe = clahe.apply(img)
plt.hist(res_clahe.ravel(), 256)
plt.suptitle("clahe")
plt.show()
res = np.hstack((img, equ, res_clahe))
cv_show('img, equ, clahe', res)
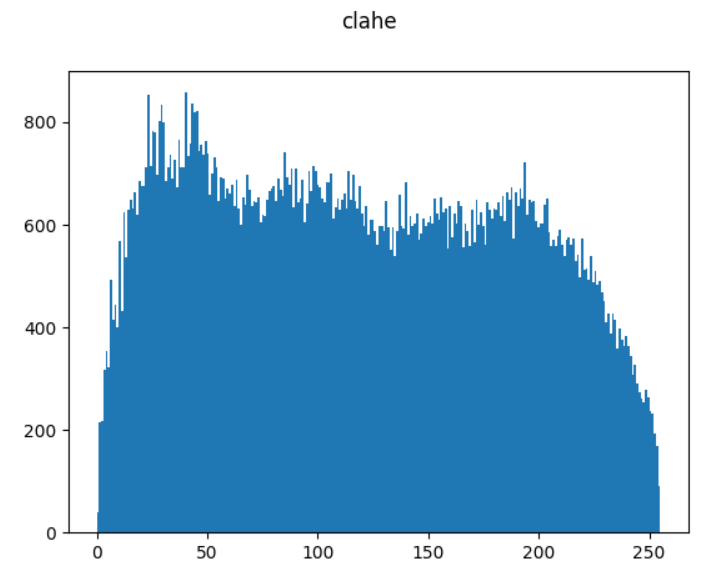
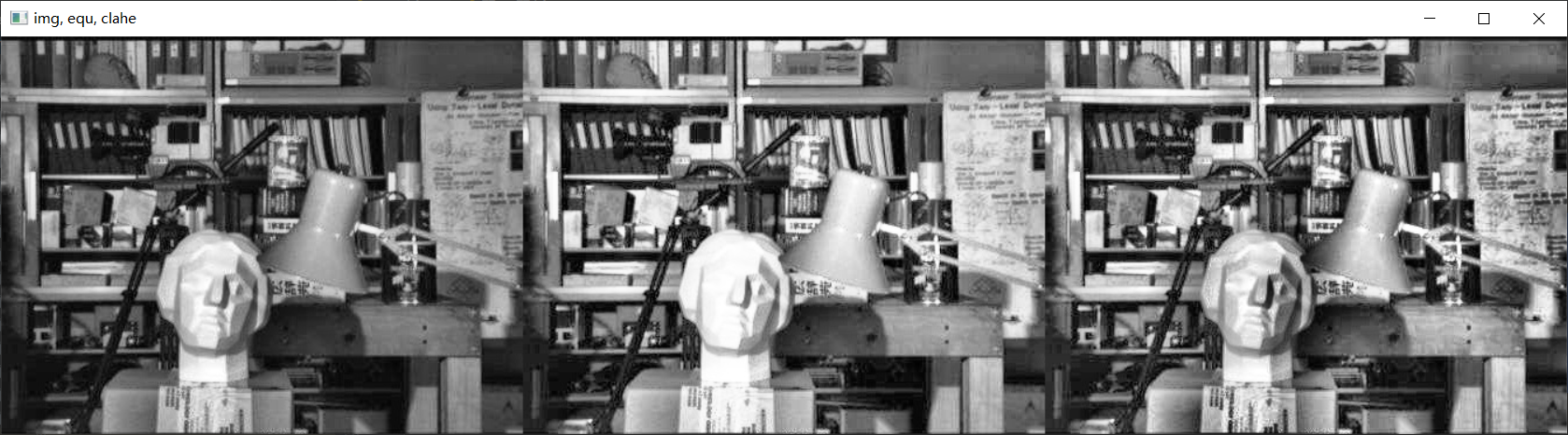
傅里叶变换
参阅:https://zhuanlan.zhihu.com/p/19763358
- 高频:变化剧烈的灰度分量,例如边界
- 低频:变化缓慢的灰度分量,例如一片大海
- 低通滤波器:只保留低频,会使得图像模糊
- 高通滤波器:只保留高频,会使得图像细节增强
图像的频谱
img = cv2.imread('media/lena.jpg', 0)
img_float32 = np.float32(img)
dft = cv2.dft(img_float32, flags=cv2.DFT_COMPLEX_OUTPUT)
dft_shift = np.fft.fftshift(dft)
magnitude_spectrum = 20 * np.log(cv2.magnitude(dft_shift[:, :, 0], dft_shift[:, :, 1]))
plt.subplot(121), plt.imshow(img, cmap='gray')
plt.title('Input Image'), plt.xticks([]), plt.yticks([])
plt.subplot(122), plt.imshow(magnitude_spectrum, cmap='gray')
plt.title('Magnitude Spectrum'), plt.xticks([]), plt.yticks([])
plt.show()
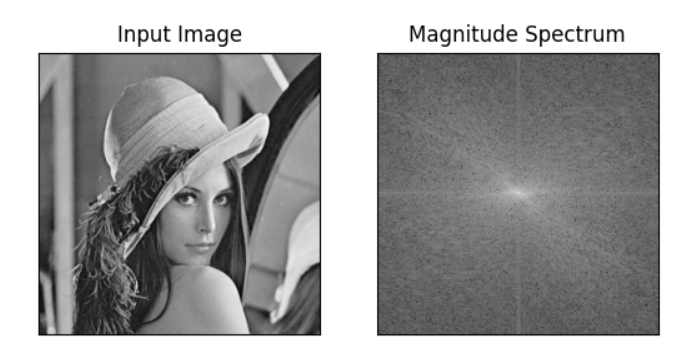
右边的图像便是 lena.jpg
的频域表示,即频谱。
中间亮的部分是低频,四周是高频。
有了频谱图,就可以方便地使用掩膜来进行高 / 低通滤波了。
高 / 低通滤波
低通滤波:
rows, cols = img.shape
crow, ccol = int(rows / 2), int(cols / 2) # 中心位置
mask = np.zeros((rows, cols, 2), np.uint8)
mask[crow - 30:crow + 30, ccol - 30:ccol + 30] = 1
fshift = dft_shift * mask
f_ishift = np.fft.ifftshift(fshift)
img_back = cv2.idft(f_ishift)
img_back = cv2.magnitude(img_back[:, :, 0], img_back[:, :, 1])
plt.subplot(121), plt.imshow(img, cmap='gray')
plt.title('Input Image'), plt.xticks([]), plt.yticks([])
plt.subplot(122), plt.imshow(img_back, cmap='gray')
plt.title('Result'), plt.xticks([]), plt.yticks([])
plt.show()
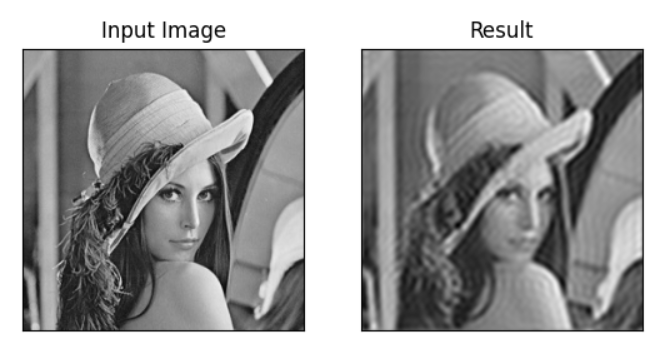
高通滤波无非就是掩膜与低通的正相反:
mask = np.ones((rows, cols, 2), np.uint8)
mask[crow - 30:crow + 30, ccol - 30:ccol + 30] = 0
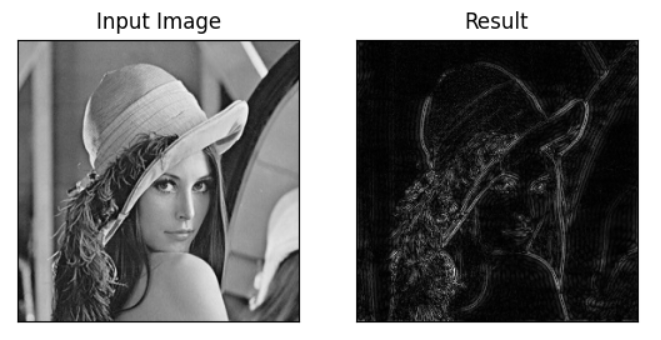